EEB student's work about getting data to do more for biodiversity
Michigan State University ecologists have developed a mathematical framework that could help monitor and preserve biodiversity without breaking the bank.
This framework or model takes low-cost data about relatively abundant species in a community and uses it to generate valuable insights on their harder-to-find neighbors. The journal Conservation Biology published the research as an Early View article on Aug. 25.
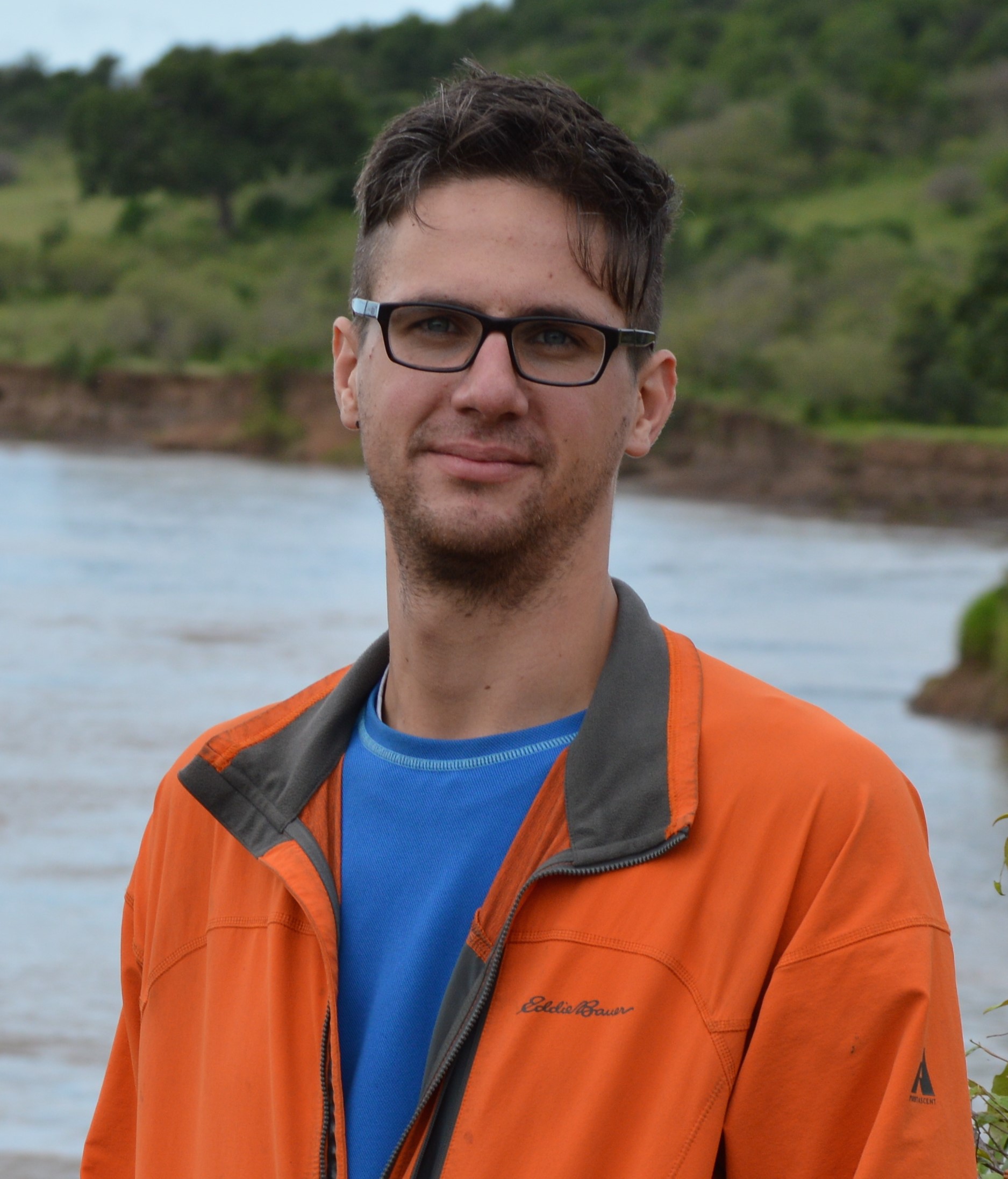
“One of the biggest challenges in monitoring biodiversity is that the species you’re most concerned about tend to be lowest in abundance or they’re the hardest species to observe during data collection,” said Matthew Farr, the lead author on the new report. “This model can be really helpful for those rare and elusive species.”
Farr, now a postdoctoral researcher at the University of Washington, helped develop the model as a doctoral student in Elise Zipkin’s Quantitative Ecology Lab in the College of Natural Science at MSU.
“There are a lot of species in the world and many of them are data deficient,” said Zipkin, an associate professor of integrative biology and director of MSU’s Ecology, Evolution and Behavior Program, or EEB. “We’re developing approaches to more quickly estimate what’s going on with biodiversity, which species are in trouble and where, spatially, do we need to focus our conservation efforts.”
After validating the model with an assist from forest-dwelling antelope in Africa, the researchers say it could be applied to a variety of other animals that meet certain criteria.
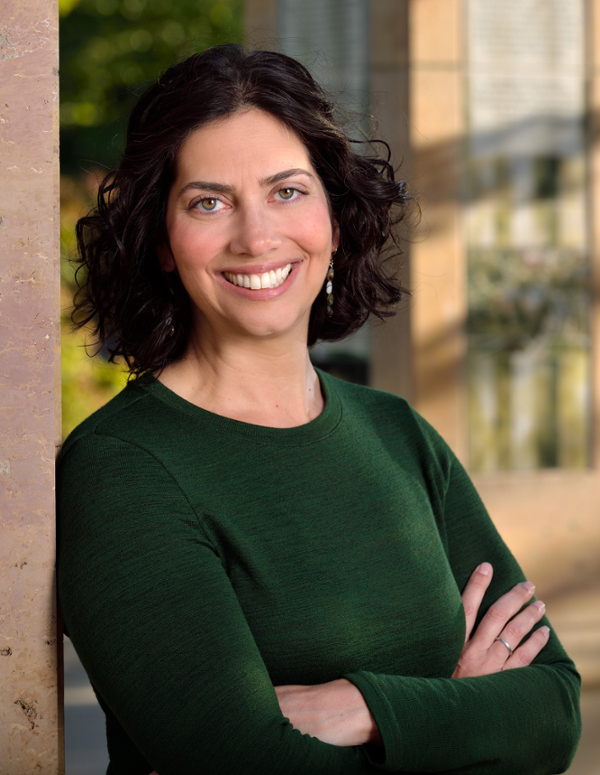
“The model doesn’t work for all types of species. It’s not a panacea,” Zipkin said. “But when it does work for a community, we can learn a lot more about member species without much data.”
For its newest model, Zipkin’s team focused on what’s called detection-nondetection data that tracks whether or not a given animal is detected in a given habitat, which typically only allows for estimation of species occurrence or distribution patterns.
“It’s basically the cheapest data and the easiest to collect,” Zipkin said. “You go to a spot, wait and see what animals are there and only need to record which species are seen.”
Researchers gather this data visually in person or with low-cost, motion-detecting camera traps that snap photos when triggered by an animal. Researchers then analyze the photos to record detection-nondetection data over time.
There are trade-offs, though. Although relatively cheap and easy to collect, detection-nondetection data doesn’t provide as much information as researchers and conservationists want. Historically, that has required intensive observational approaches such as tagging and tracking animals.
“Intensive tracking lets us calculate all sorts of things about animals and their communities, but those data are expensive and hard to get,” Zipkin said. “For certain species, it’s impossible.”
The MSU team realized that, for the right animals, they could use an understanding of animal behavior and statistics to close the information gap by squeezing more insight out of detection-nondetection data.
“For some species, these are the best data you can get,” Farr said. “Now we can get more out of it. Our model allows for estimation of abundance trends, reproduction rates and species survival probabilities — all using only detection-nondetection data.”
That may sound like magic — some of Zipkin’s colleagues have even said so — but there’s nothing supernatural about the model. Like much of science, it’s the result of hard work, collaboration and building on previous efforts in the field.